遥感大影像位深转8bit的方法及实现
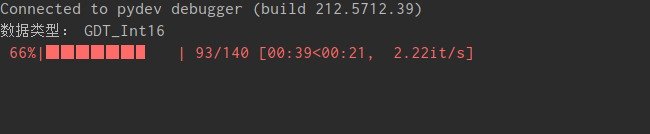
遥感大影像位深转8bit的方法及实现
ytkz位深
位深(Bit Depth)是指数字图像中每个像素用于记录颜色信息的位数。位深越高,可用于表示颜色的信息就越丰富,图像的颜色就越真实且细腻。然而,位深越高,图像文件的大小也就越大。
在数字图像中,常见的位深有8位、16位、24位和32位等。下面我们来看一下8位和16位位深的区别:
- 8位位深:每个像素用8位(即1字节)来记录颜色信息。在灰度图像中,8位位深可以表示256(即$2^8)种不同的灰度等级。在彩色图像中,通常每种颜色(红、绿、蓝)都用8位来记录,因此可以表示256^3(即16777216)种不同的颜色。
- 16位位深:每个像素用16位(即2字节)来记录颜色信息。在灰度图像中,16位位深可以表示65536(即$2^{16})种不同的灰度等级。在彩色图像中,通常每种颜色(红、绿、蓝)都用16位来记录,因此可以表示65536^3种不同的颜色。
从这里可以看出,16位位深的图像比8位位深的图像有更丰富的颜色信息,能更精细地表示图像的颜色变化。然而,16位位深的图像文件的大小也会比8位位深的图像文件大一倍。
遥感影像大多数未拉伸前,一般为10\14\16bit。
为什么要做
在深度学习中,数据的表示范围和精度对训练的影响是一个重要的考虑因素。虽然16位(即2字节)的数据可以提供更大的范围和更高的精度,但这也意味着需要更多的计算资源(如内存和计算能力)。在许多情况下,8位(即1字节)的数据已经足够满足深度学习的需求,并且可以显著减少所需的计算资源。
以下是将16位数据转换为8位数据的一些主要原因:
- 内存和存储空间:8位数据只需要16位数据一半的内存和存储空间。这对于处理大量数据(如图像或视频)的深度学习任务来说是非常重要的。
- 计算速度:处理8位数据的计算速度通常会比处理16位数据更快。这是因为计算机的内存和处理器都是按字节(8位)组织的,处理8位数据可以更有效地利用这些硬件资源。
- 兼容性:许多深度学习框架和算法都是针对8位数据进行优化的。使用8位数据可以确保与这些框架和算法的兼容性。
- 精度需求:虽然16位数据可以提供更高的精度,但在许多深度学习任务中,这种高精度并不是必需的。实际上,一些研究表明,使用较低精度的数据对深度学习模型的性能影响很小。
总的来说,将16位数据转换为8位数据是一种权衡。这种转换可能会损失一些精度,但可以获得更高的计算效率和更好的兼容性。
我做16位降8位的原因大多数源自于深度学习训练模型需要8bit的需求。
有什么困难
常规的16bit转8bit,是利用拉伸的方式进行。但是大影像进行降位处理会出现内存不足的问题。就是不能把数据一次性储存到内存中。一般会出现以下错误提示:
numpy.core._exceptions.MemoryError: Unable to allocate 4.14 GiB for an array with shape (4, 29648, 38489) and data type uint8
这个错误信息表示程序试图在内存中创建一个非常大的数组,但是可用的内存不足,因此无法完成这个操作。
怎么解决
利用分块的思路,按照规则依次把小分块进行拉伸,然后保存到新的tif中。
其中首先计算了整个图像的最大值和最小值,然后在处理每个分块时使用这些参数。
这样做的好处在于,分块处理拉伸问题,但全局使用同样的参数,使得最后结果无明显的分块痕迹。
具体代码
#!/usr/bin/env python
# -*- coding: utf-8 -*-
# @Time : 2024/2/27 21:40
# @File : 16to8.py
from osgeo import gdal
import numpy as np
import time
import os
from tqdm import tqdm
class ImageCompressor:
def __init__(self, path):
self.path = path
def read_img(self, input_file):
'''
这个方法用于打开输入的图像文件,并返回一个包含图像数据和图像的行数、列数、波段数的元组。
:param input_file: 输入的图像文件
:return: 包含图像数据和图像的行数、列数、波段数的元组
'''
in_ds = gdal.Open(input_file, gdal.GA_ReadOnly) # 仅打开文件
rows = in_ds.RasterYSize # 获取数据高度
cols = in_ds.RasterXSize # 获取数据宽度
bands = in_ds.RasterCount # 获取数据波段数
datatype = in_ds.GetRasterBand(1).DataType
# GDT_Byte = 1, GDT_UInt16 = 2, GDT_UInt32 = 4, GDT_Int32 = 5, GDT_Float32 = 6
data_type_name = {1: 'GDT_Byte', 2: 'GDT_UInt16',3:'GDT_Int16', 4: 'GDT_UInt32', 5: 'GDT_Int32', 6: 'GDT_Float32'}
print("数据类型:", data_type_name[datatype])
array_data = in_ds.ReadAsArray() # 将数据写成数组,读取全部数据,numpy数组
del in_ds
return array_data, rows, cols, bands
def write_img(self, read_path, img_array):
'''
这个方法用于将图像数据写入到一个新的文件中。
:param read_path: 读取的文件路径
:param img_array: 图像数据
:return: None
'''
read_pre_dataset = gdal.Open(read_path)
img_transf = read_pre_dataset.GetGeoTransform() # 仿射矩阵
img_proj = read_pre_dataset.GetProjection() # 地图投影信息
print("read shape:", img_array.shape, img_array.dtype.name)
if 'uint8' in img_array.dtype.name:
datatype = gdal.GDT_Byte
elif 'int16' in img_array.dtype.name:
datatype = gdal.GDT_UInt16
else:
datatype = gdal.GDT_Float32
if len(img_array.shape) == 3:
img_bands, im_height, im_width = img_array.shape
else:
img_bands, (im_height, im_width) = 1, img_array.shape
filename = read_path[:-4] + '_unit8' + ".tif"
driver = gdal.GetDriverByName("GTiff") # 创建文件驱动
dataset = driver.Create(filename, im_width, im_height, img_bands, datatype)
dataset.SetGeoTransform(img_transf) # 写入仿射变换参数
dataset.SetProjection(img_proj) # 写入投影
if img_bands == 1:
dataset.GetRasterBand(1).WriteArray(img_array)
else:
for i in range(img_bands):
dataset.GetRasterBand(i + 1).WriteArray(img_array[i])
def compress(self, origin_16, chunk_size=5000):
'''
这个方法是图像压缩的主要部分。它首先读取原图像的数据,然后计算全局的最大值和最小值,然后创建一个新的文件,
并在每个分块上应用线性拉伸,最后将处理后的分块写入到新文件中。
:param origin_16: 原图像路径
:param chunk_size: 分块大小
:return: None
'''
def linear_stretching(img, min, max):
'''
@todo 线性拉伸
@param img: 图像 二维数组
@return: 拉伸后的图像
'''
img = np.array(img)
img = (img - min) / (max - min) * 255
return img
array_data, rows, cols, bands = self.read_img(origin_16)
global_min = np.min(array_data)
global_max = np.max(array_data)
read_pre_dataset = gdal.Open(origin_16)
img_transf = read_pre_dataset.GetGeoTransform() # 仿射矩阵
img_proj = read_pre_dataset.GetProjection() # 地图投影信息
filename = origin_16[:-4] + '_unit8' + ".tif"
driver = gdal.GetDriverByName("GTiff") # 创建文件驱动
dataset = driver.Create(filename, cols, rows, bands, gdal.GDT_Byte)
dataset.SetGeoTransform(img_transf) # 写入仿射变换参数
dataset.SetProjection(img_proj) # 写入投影
total_chunks = (rows // chunk_size + 1) * (cols // chunk_size + 1) * bands
with tqdm(total=total_chunks) as pbar:
for i in range(bands):
for start_row in range(0, rows, chunk_size):
end_row = start_row + chunk_size if start_row + chunk_size < rows else rows
for start_col in range(0, cols, chunk_size):
end_col = start_col + chunk_size if start_col + chunk_size < cols else cols
chunk = array_data[i, start_row:end_row, start_col:end_col]
stretched_chunk = linear_stretching(chunk, global_min, global_max)
dataset.GetRasterBand(i + 1).WriteArray(stretched_chunk, xoff=start_col, yoff=start_row)
pbar.update()
dataset = None
def listdir(self, path, list_name):
'''
用于获取指定路径下所有符合指定格式的文件的列表
:param path: 文件路径
:param list_name: 用于存储文件名的列表
:return: 返回文件名列表
'''
panduan_geshi = [".tif", ".pix", ".img", ]
for file in os.listdir(path):
file_path = os.path.join(path, file)
if os.path.isdir(file_path):
self.listdir(file_path, list_name)
elif os.path.isfile(file_path):
if os.path.splitext(file_path)[1] in panduan_geshi:
list_name.append(file_path)
else:
print("File Error")
def isfile(self, path):
'''
判断是否是文件
'''
if os.path.isfile(path):
return True
else:
return False
def ispath(self, path):
'''
判断是否是路径
'''
if os.path.isdir(path):
return True
else:
return False
def process(self):
'''
整个类的入口点。它首先检查给定的路径是文件还是目录,然后对每个文件调用 compress 方法进行处理。
'''
if self.ispath(self.path):
lisst = []
self.listdir(self.path, lisst)
print(len(lisst))
for file_name in lisst:
print(file_name)
start = time.time()
self.compress(file_name)
print("cost time:", time.time() - start)
if self.isfile(self.path):
start = time.time()
self.compress(self.path)
print("cost time:", time.time() - start)
if __name__ == '__main__':
path = r"D:\\GF2.tif"
compressor = ImageCompressor(path)
compressor.process()
代码解析
这段代码是一个名为ImageCompressor
的类,主要用于处理大型地理信息系统(GIS)图像数据,并将16位数据压缩为8位数据。它使用了gdal
库来读取和写入图像,numpy
库来处理图像数据,以及tqdm
库来显示处理进度。以下是对这段代码主要部分的解释:
__init__
函数:初始化函数,接收一个路径参数。read_img
函数:使用gdal
库打开指定路径的图像文件,读取图像的大小、波段数和数据类型,并将图像数据读取为numpy
数组。write_img
函数:根据输入的图像路径和数组,使用gdal
库创建一个新的图像文件,并将数组数据写入新文件。如果输入数组的数据类型是8位无符号整数,新文件的数据类型将设置为GDT_Byte
;如果是16位整数,将设置为GDT_UInt16
;否则,将设置为GDT_Float32
。compress
函数:这是主要的图像处理函数。它首先读取原始16位图像数据,然后对图像进行线性拉伸,将数据范围从全局最小值和最大值拉伸到0和255。然后,它将处理后的8位数据写入新的图像文件。这个函数使用了块处理策略,将图像分割成多个小块进行处理,以降低内存使用。listdir
函数:递归地列出指定路径下所有的.tif, .pix, .img文件。isfile
和ispath
函数:检查指定路径是文件还是目录。process
函数:如果输入路径是目录,它将处理目录下的所有图像文件;如果输入路径是文件,它将只处理这个文件。在处理每个文件之前和之后,它都会打印当前时间,以便计算处理时间。
这个类需要在实例化后调用process
方法来开始处理图像,例如:
compressor = ImageCompressor("/path/to/images")
compressor.process()
这将处理指定路径下的所有.tif, .pix, .img文件,并将处理后的图像保存为新的.tif文件。
改进
把int16—>int8拓展为任意类型—>int8
把拉伸类型从线性拉伸改为 百分比线性拉伸
具体代码如下:
#!/usr/bin/env Python
# coding=utf-8
# @Time : 2024/2/26 08:40
# @File : 16to8.py
from osgeo import gdal
import numpy as np
import time
import os
from tqdm import tqdm
class ImageCompressor:
def __init__(self, path):
self.path = path
def read_img(self, input_file):
'''
这个方法用于打开输入的图像文件,并返回一个包含图像数据和图像的行数、列数、波段数的元组。
:param input_file: 输入的图像文件
:return: 包含图像数据和图像的行数、列数、波段数的元组
'''
in_ds = gdal.Open(input_file, gdal.GA_ReadOnly) # 仅打开文件
rows = in_ds.RasterYSize # 获取数据高度
cols = in_ds.RasterXSize # 获取数据宽度
bands = in_ds.RasterCount # 获取数据波段数
datatype = in_ds.GetRasterBand(1).DataType
# GDT_Byte = 1, GDT_UInt16 = 2, GDT_UInt32 = 4, GDT_Int32 = 5, GDT_Float32 = 6
data_type_name = {1: 'GDT_Byte', 2: 'GDT_UInt16',3:'GDT_Int16', 4: 'GDT_UInt32', 5: 'GDT_Int32', 6: 'GDT_Float32', 7:'GDT_CFloat64'}
print("数据类型:", data_type_name[datatype])
array_data = in_ds.ReadAsArray() # 将数据写成数组,读取全部数据,numpy数组
del in_ds
return array_data, rows, cols, bands
def write_img(self, read_path, img_array):
'''
这个方法用于将图像数据写入到一个新的文件中。
:param read_path: 读取的文件路径
:param img_array: 图像数据
:return: None
'''
read_pre_dataset = gdal.Open(read_path)
img_transf = read_pre_dataset.GetGeoTransform() # 仿射矩阵
img_proj = read_pre_dataset.GetProjection() # 地图投影信息
print("read shape:", img_array.shape, img_array.dtype.name)
if 'uint8' in img_array.dtype.name:
datatype = gdal.GDT_Byte
elif 'int16' in img_array.dtype.name:
datatype = gdal.GDT_UInt16
else:
datatype = gdal.GDT_Float32
if len(img_array.shape) == 3:
img_bands, im_height, im_width = img_array.shape
else:
img_bands, (im_height, im_width) = 1, img_array.shape
filename = read_path[:-4] + '__unit8' + ".tif"
driver = gdal.GetDriverByName("GTiff") # 创建文件驱动
dataset = driver.Create(filename, im_width, im_height, img_bands, datatype)
dataset.SetGeoTransform(img_transf) # 写入仿射变换参数
dataset.SetProjection(img_proj) # 写入投影
# 无效值为0
if img_bands == 1:
dataset.GetRasterBand(1).WriteArray(img_array)
else:
for i in range(img_bands):
dataset.GetRasterBand(i + 1).WriteArray(img_array[i])
def compress(self, origin_16, chunk_size=5000):
'''
这个方法是图像压缩的主要部分。它首先读取原图像的数据,然后计算全局的最大值和最小值,然后创建一个新的文件,
并在每个分块上应用线性拉伸,最后将处理后的分块写入到新文件中。
:param origin_16: 原图像路径
:param chunk_size: 分块大小
:return: None
'''
def linear_stretching(img, min, max):
'''
@todo 线性拉伸
@param img: 图像 二维数组
@return: 拉伸后的图像
'''
img = np.array(img)
img = (img - min) / (max - min) * 255
return img
array_data, rows, cols, bands = self.read_img(origin_16)
global_min = np.min(array_data)
global_max = np.max(array_data)
global_min = np.percentile(array_data, 2)
global_max = np.percentile(array_data, 96)
if 'float64' in global_min.dtype.name:
global_min = 0
read_pre_dataset = gdal.Open(origin_16)
img_transf = read_pre_dataset.GetGeoTransform() # 仿射矩阵
img_proj = read_pre_dataset.GetProjection() # 地图投影信息
filename = origin_16[:-4] + '_unit8' + ".tif"
driver = gdal.GetDriverByName("GTiff") # 创建文件驱动
dataset = driver.Create(filename, cols, rows, bands, gdal.GDT_Byte)
dataset.SetGeoTransform(img_transf) # 写入仿射变换参数
dataset.SetProjection(img_proj) # 写入投影
total_chunks = (rows // chunk_size + 1) * (cols // chunk_size + 1) * bands
with tqdm(total=total_chunks) as pbar:
for i in range(bands):
for start_row in range(0, rows, chunk_size):
end_row = start_row + chunk_size if start_row + chunk_size < rows else rows
for start_col in range(0, cols, chunk_size):
end_col = start_col + chunk_size if start_col + chunk_size < cols else cols
chunk = array_data[i, start_row:end_row, start_col:end_col]
stretched_chunk = linear_stretching(chunk, global_min, global_max)
stretched_chunk[stretched_chunk == 0 ] = np.nan
stretched_chunk_uint8 = stretched_chunk.astype(np.uint8)
dataset.GetRasterBand(i + 1).WriteArray(stretched_chunk_uint8, xoff=start_col, yoff=start_row)
pbar.update()
dataset = None
def listdir(self, path, list_name):
'''
用于获取指定路径下所有符合指定格式的文件的列表
:param path: 文件路径
:param list_name: 用于存储文件名的列表
:return: 返回文件名列表
'''
panduan_geshi = [".tif", ".pix", ".img", ]
for file in os.listdir(path):
file_path = os.path.join(path, file)
if os.path.isdir(file_path):
self.listdir(file_path, list_name)
elif os.path.isfile(file_path):
if os.path.splitext(file_path)[1] in panduan_geshi:
list_name.append(file_path)
else:
print("File Error")
def isfile(self, path):
'''
判断是否是文件
'''
if os.path.isfile(path):
return True
else:
return False
def ispath(self, path):
'''
判断是否是路径
'''
if os.path.isdir(path):
return True
else:
return False
def process(self):
'''
整个类的入口点。它首先检查给定的路径是文件还是目录,然后对每个文件调用 compress 方法进行处理。
'''
if self.ispath(self.path):
lisst = []
self.listdir(self.path, lisst)
print(len(lisst))
for file_name in lisst:
print(file_name)
start = time.time()
self.compress(file_name)
print("cost time:", time.time() - start)
if self.isfile(self.path):
start = time.time()
self.compress(self.path)
print("cost time:", time.time() - start)
if __name__ == '__main__':
path = r"clip3.tif"
compressor = ImageCompressor(path)
compressor.process()